Technology
AI and design:
Competing with the machines
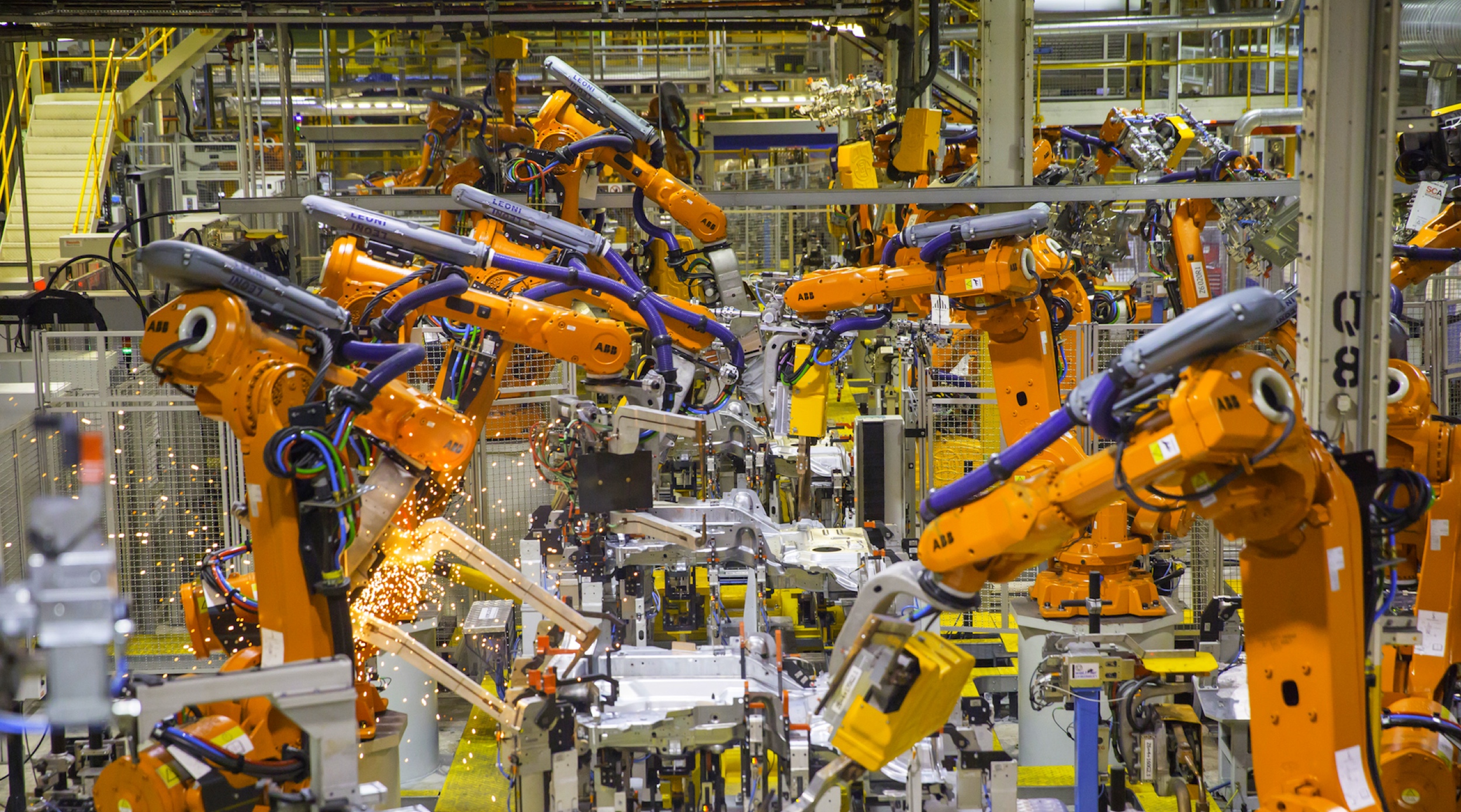
The word robot was coined by Czech author Karel Čapek, who used it in his 1921 play R.U.R. to describe a fleet of intelligent machines. He named his invention based on the Slavonic word “rabota,” which means labor. More tellingly, rabota is also the root of the word “rob” or slave. And in fact, automation promises to get away with some of the most tedious, repetitive tasks we love to hate.
Take telemarketing for example, one of the most slave-like occupations in the 21st Century. I think, it is safe to assume that no one has ever dreamt of a career in telemarketing or would miss it if it were to disappear. Luckily for us, it is the job most likely to disappear, according to Carl Benedikt Frey’s and Michael A. Osborne’s excellent study The Future of Employment: How Susceptible Are Jobs to Computerization. They examined some 702 occupations and evaluated the level of risk for each one of them.
The extensive list and ranking is interesting in an of itself (you may want to consider a career in recreational therapy. Whatever that is — it is least threatened.) More importantly, the study identifies three factors that impact that level of risk, allowing us to start thinking about how automation will impact our own specific fields. These three criteria are: perception and manipulation, creative intelligence, and social intelligence. I’ve added to that framework the concept of predictability. In a nutshell, the occupations that are most likely to resist automation would:
1. involve complex perception and manipulation activities,
2. require the ability to come up with clever ideas, or new ways of solving problems,
3. involve negotiation, persuasion, empathy and caring,
4. demand the individual to be highly adaptable to different contexts and situations
My interest is not only in exploring the topic of automation broadly, but looking at how these apply to various design professions. A quick examination of the above list and you might think that since that sounds a lot like a designer’s skill set, you must be safe. The reality is a bit more complex, so let’s take a look at each factors and relate it back to design professions specifically.
Perception and manipulation
This class of automation challenges begins with perception systems mostly related to 2D computer vision and 3D spatial sensing technologies — for example, jobs that involve simply recognizing objects or aspects of objects through jobs that involve lots of fine finger dexterity or precise manipulation of small irregular objects. At a higher level, manual dexterity tasks combine the challenges of micro-manipulation with broader physical movements, like opening a door, climbing stairs, or stacking a set of boxes. If you combine these two tasks and add in the challenge of working in tight physical spaces, then you have a job that is highly resilient to displacement. Plumbing is one such example. Another, less obvious one is car assembly lines — while huge robotic equipment seems to do most of the work, you still see humans doing various micro-manipulation tasks inside the cramped, half-built vehicles.
For designers, visual perception is a critical skill and for those crafting 3D objects, so is spatial manipulation. So you might quickly conclude that if computers aren’t yet good at these things then we are safe, and you’d be partly right. Yet in the last few years, computer vision and deep learning systems have been deconstructing massive datasets of 2D images and 3D objects, working to inform a algorithmic designer as to ways to manipulate compositions, form, texture, etc.
Take the Prisma app, for example — our Sunday night dinners and backyard parties can suddenly look like a Van Gough masterpiece, not by design, but by data. Tools like that will disrupt the illustration profession, potentially reducing hours of laborious craft into software processing that spits out image compositions of any objects, in any art style.
In web page design we have the controversial (at least in the design community) Grid.io, which in some cases appears to do photographic and typographic composition based on high level parameters set by the designer. The combination of deep learning systems with simple codified heuristics, rules, best practices and principles means that many of the perceptual activities that a human designer thinks of as their idiosyncratic “eye and craft,” will be replaceable by AI systems sooner or later.
As for 3D manipulation, 3D printing techniques are making it possible to create physical objects far beyond the capability of even the best human craftsman.
Creative intelligence
The second area that is hard for AI technologies is creative intelligence. This is the ability to come up with valuable ideas and figure out ways to solve different kinds of problems.
In a recent piece, I shared thoughts on the nature of creativity, or the ability to create ideas or artifacts that are novel and valuable. By dividing creativity this way, it becomes clear that ascribing value (in the broadest sense) to an idea is fundamentally a subjective assessment. Value changes in context, culture across time, and with different individual identities and personalities. It’s why attitudes and preferences for art, music, fashion, vary so greatly.
In design, a valuable or clever idea may also be the result of a particular insight or perspective on behalf of the team. That breakthrough may result from rigorous research and understanding, or simply from social perspective, philosophical bias or what is commonly identified as individual “genius” or “talent.” Ideas are extremely resistant to codification. In their early form they are slippery, elusive and often feel good for seemingly emotional reasons. As many design thinkers have reflected, great design is as much about the hunt for the perfect framing question, as it is about coming up with a valuable answer, which makes the challenge of codifying valuable ideation even tougher.
Advances in overall computing power have made simulation possible so that similar parameter driven designs for objects and buildings can be created and millions of variations evolved to find the optimal engineering tradeoffs. This has been termed Generative design, and its been around for 30 or so years in various research facilities. Autodesk Dreamcatcher has been on the forefront of demonstrating the early proof of concept of this idea.
Tools like Dreamcatcher make creating novel solutions, an aspect of creative intelligence, highly susceptible to automation. Especially in the visual realm, there are many known techniques that can be applied to the creation of novelty. Computers armed with a reasonable understanding of goals can simply create massive numbers of design variations, remixing content, techniques, principles and patterns infinitely. They can also analyze the technique and emulate it, when sufficient example data exists.
Focusing only on creating novelty, however, is fake creative intelligence. While machine intelligences will be an increasingly powerful tool, it seems hard to imagine computers spontaneously challenging themselves with creative questions, generating creative solutions and evaluating the value of those solutions to find something optimal. To quote Picasso “[Computers] but they are useless. They can only give you answers.” Something that is perhaps inherently human is the ability to formulate the right question.
Social intelligence
Social intelligence is about real time recognition of human emotions. It poses a hard to overcome set of problems related to the complexity of codifying human social, cultural and emotional behavior. Codifying “common sense” and how senses and experience inform an awareness of the dynamics of situations, emotions, behaviors and contexts is hard to program. Examples range from the most basic kinds of understanding and prediction of physical interactions, like playing Jenga, to subtle cultural norms, like etiquette, politeness, taboo, political correctness. It also spans understanding human intent, motivations, emotions and actions. From an engineering perspective, these are all discrete and difficult challenges.
One relevant test of computers’ ability to do this is the Turing Test. Devised by Alan Turing in 1950, it was intended to test progress in AI technology to see if computers could fool a human into thinking it was communicating with another human in text based communications. In 2014, in an annual Turing Test, Eugene Goostman, a chatbot pretending to be a 13 year old Ukrainian boy, convinced a third of the judges that it was a real human. (Since Eugene claimed he was a tween, with English his second language, he was able to slide by with some communication wrinkles.)
Yet, despite the progress that is being made, jobs that require high social intelligence, like public relations, acting, comedy writing seem unlikely to be replaced by automation in the near future.
Similarly, social intelligence is at the core of human centered design. Most designers would agree that great designers possess high social intelligence, and a great understanding of culture and humanity. Furthermore, as we are called on to design for richer cross-disciplinary contexts, we are almost constantly in a state of negotiation and persuasion.
Predictability
Predictable tasks are not just those that are repeated in some endless process loop. If your job is something that can be broken down into a flow chart, with a series of decisions and actions from start to finish, then your job is potentially highly susceptible to automation. As long as the nature of the decision-making and action in each step doesn’t involve complex perception and manipulation, creative intelligence, or social intelligence, automation is probably right around the corner.
Take for example an autonomous car navigating through city traffic, from one destination to another. On the surface, this would appear to be highly complex in terms of predictability. However, from a logic perspective, this problem is more solvable. There is a clear path-finding problem to solve (how to get there using detailed maps of roads), there are unambiguous rules of the road and there are easily measurable environmental obstacles to recognize, understand and react to. Only this last category — the perception and reaction to environmental obstacles — involves complex perception activities. At a complex intersection with multiple lanes, pedestrians, bike lanes, even random debris, traffic signals, things obscured by other vehicles — the perception challenge is quite formidable. To make matters more technically complex, the system must not just be “locating” the positions of these things but “predicting” the movement of these objects relative to the physics of the vehicle. Whilst this sounds and is daunting, the basic decision making and actions the car has to operate by are still very simple — try to avoid hitting anything whilst obeying the rules of the road, and getting the car from A-B.
Different design professions are rooted in different traditions and different degrees of adherence to elaborate creative processes and more systematic, predictable methods. On one hand, many design professions can be broken into a series of steps. On the other, it’s the content of each of the decision-making steps that determines the degree of susceptibility to automation. If we examine brainstorming, one step that we use in almost every design process, we can see the important role creative and social intelligence play and how they make predictability virtually impossible.
Competing with the machines:
Can we win the battle?
Of the four criteria, social intelligence and creative Intelligence stand out as the most difficult areas for computer science researchers to make near term progress that would be good enough to displace significant numbers of jobs in the 5–15 year timeframe.
Entrepreneurs (and designers) in the AI field are busy right now hunting for parts of occupations or whole occupations that have relatively straightforward predictability scenarios. There are hundreds of examples of jobs that look highly susceptible to displacement from secretarial positions to tax preparation, insurance policy clerks, insurance underwriters, data entry clerks, loan officers, credit analysts, book keeping accounting, shipping and receiving clerks, office administrators and hundreds more. Over the next ten years we will increasingly feel the impact of this work as we witness somewhat unprecedented occupation displacement through increasing automation and robotics.
While there are attempts to automate certain perceptual and novelty aspects of creativity and design, for the next few decades, I think we can conclude that most design professions are probably reasonably safe from computerization. But before we exhale a collective sigh of relief, let’s be realistic — our professions are going to be deeply impacted by AI.